Robin Duraz
Directeur(s): Sandrine Vaton
Encadrant(s): David Espès & Julien Francq
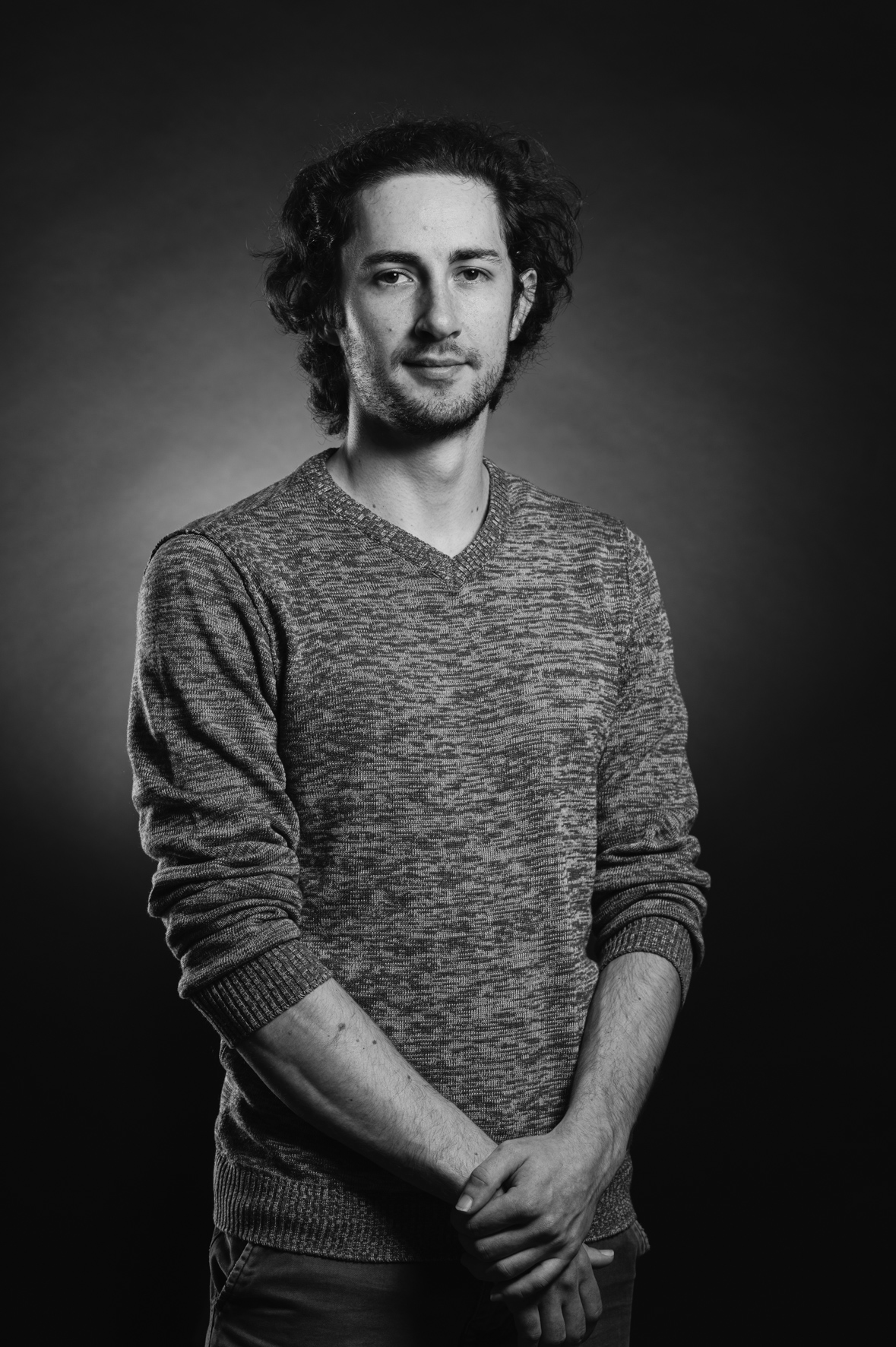
SUJET DE THÈSE
Machine Learning Analysis for Cyber Attack Detection and Classification on Board Ships
Work Summary
The digitization of onboard environments is becoming increasingly prevalent in the maritime sector, leading to the convergence of Information Technology (IT) and Operational Technology (OT) within naval vessels. This evolution mirrors the architecture of the IT/OT integration seen in modern industrial systems. To ensure the security of naval vessels, it is crucial to detect cyber-attacks that could compromise their proper functioning. Various types of attacks need to be considered, including vertical attacks originating from higher system levels and attacks targeting the availability of operational systems, which could be particularly critical during a mission.
Traditionally, onboard security components (e.g., intrusion detection probes) have relied on static security rules. This approach presents several limitations, such as the need for frequent rule updates and an inability to detect novel attacks (zero-day attacks).
This thesis aims to enhance the security of naval vessels by leveraging machine learning techniques to analyze metrics collected at various levels within the IT/OT system. The objective is to automate the detection and classification of cyber-attacks or other system malfunctions. A particular focus will be placed on semi-supervised learning methods, where only partial labels are available for associating diagnostics with observations.